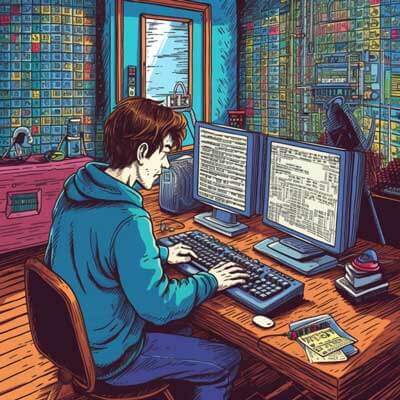
Table of Contents
Overview of Averaging Functions in Python
When working with data in Python, calculating averages is a common task. Whether you're analyzing a dataset, performing statistical analysis, or working with numerical data in general, being able to calculate averages is essential. In Python, there are several ways to calculate averages, but one of the most useful and efficient libraries for this task is Numpy.
Numpy is a popular library in the Python ecosystem that provides support for large, multi-dimensional arrays and matrices, along with a collection of mathematical functions to operate on these arrays. In this article, we will explore how to use Numpy's averaging functions to calculate mean and average values efficiently.
Related Article: How To Access Index In Python For Loops
Calculating Mean with Numpy
The mean is a commonly used measure of central tendency that represents the average value of a dataset. Numpy provides a function called mean
that allows us to calculate the mean of an array or a specific axis of an array.
To calculate the mean of an entire array, we can simply pass the array as an argument to the mean
function. Let's consider the following example:
import numpy as np data = np.array([1, 2, 3, 4, 5]) mean_value = np.mean(data) print("Mean:", mean_value)
In this example, we create a Numpy array data
with values [1, 2, 3, 4, 5]. We then use the mean
function from Numpy to calculate the mean of the entire array, and store the result in the variable mean_value
. Finally, we print the mean value.
Output:
Mean: 3.0
As we can see, the mean of the array [1, 2, 3, 4, 5] is 3.0.
Using the Numpy Average Function
In addition to the mean
function, Numpy also provides an average
function that can be used to calculate the average of an array or a specific axis of an array. The average
function is more flexible than the mean
function, as it allows us to specify weights for the elements of the array.
To calculate the average of an entire array using the average
function, we can pass the array as an argument to the function, similar to the mean
function. Let's consider the following example:
import numpy as np data = np.array([1, 2, 3, 4, 5]) average_value = np.average(data) print("Average:", average_value)
In this example, we create a Numpy array data
with values [1, 2, 3, 4, 5]. We then use the average
function from Numpy to calculate the average of the entire array, and store the result in the variable average_value
. Finally, we print the average value.
Output:
Average: 3.0
As we can see, the average of the array [1, 2, 3, 4, 5] is also 3.0, which is the same as the mean value.
Choosing the Axis for Numpy Averaging
One of the useful features of Numpy is its ability to perform calculations along a specific axis of an array. This can be particularly useful when working with multi-dimensional arrays or when we want to calculate averages for specific subsets of the data.
When calculating averages with Numpy, we can specify the axis along which we want to perform the averaging. The axis parameter accepts an integer or a tuple of integers that specify the axis or axes along which the averaging should be performed. The default value is None
, which means the averaging will be performed over the entire array.
Let's consider an example to illustrate the concept of axis in Numpy averaging:
import numpy as np data = np.array([[1, 2, 3], [4, 5, 6]]) mean_axis_0 = np.mean(data, axis=0) mean_axis_1 = np.mean(data, axis=1) print("Mean along axis 0:", mean_axis_0) print("Mean along axis 1:", mean_axis_1)
In this example, we create a Numpy array data
with shape (2, 3) that represents a 2-dimensional array with two rows and three columns. We then use the mean
function from Numpy to calculate the mean along axis 0 and axis 1 of the array. Finally, we print the mean values along each axis.
Output:
Mean along axis 0: [2.5 3.5 4.5] Mean along axis 1: [2. 5.]
As we can see, when we calculate the mean along axis 0, the result is an array [2.5, 3.5, 4.5], which represents the mean values of each column. When we calculate the mean along axis 1, the result is an array [2.0, 5.0], which represents the mean values of each row.
Related Article: How to Force Pip to Reinstall the Current Version in Python
Applying Weights with Numpy Average
In some cases, we may want to apply weights to the elements of an array when calculating the average. Numpy's average
function allows us to do this by specifying the weights
parameter.
The weights
parameter accepts an array-like object that specifies the weight for each element of the input array. The shape of the weights
array should be compatible with the input array. If the weights
parameter is not specified, all elements are assumed to have equal weight.
Let's consider an example to illustrate how to apply weights with Numpy's average
function:
import numpy as np data = np.array([1, 2, 3, 4, 5]) weights = np.array([0.1, 0.2, 0.3, 0.2, 0.1]) weighted_average = np.average(data, weights=weights) print("Weighted Average:", weighted_average)
In this example, we create a Numpy array data
with values [1, 2, 3, 4, 5]. We also create a Numpy array weights
with values [0.1, 0.2, 0.3, 0.2, 0.1], which represent the weights for each element of the data
array. We then use the average
function from Numpy to calculate the weighted average of the data
array using the weights
array, and store the result in the variable weighted_average
. Finally, we print the weighted average value.
Output:
Weighted Average: 2.9
As we can see, the weighted average of the array [1, 2, 3, 4, 5] with the specified weights [0.1, 0.2, 0.3, 0.2, 0.1] is 2.9.
Averaging Multiple Arrays with Numpy
Numpy allows us to calculate the average of multiple arrays using its averaging functions. We can pass multiple arrays as arguments to the mean
or average
functions, and Numpy will perform the averaging operation across the corresponding elements of the arrays.
Let's consider an example to illustrate how to average multiple arrays with Numpy:
import numpy as np array1 = np.array([1, 2, 3]) array2 = np.array([4, 5, 6]) mean_arrays = np.mean([array1, array2], axis=0) print("Mean of Arrays:", mean_arrays)
In this example, we create two Numpy arrays array1
and array2
with values [1, 2, 3] and [4, 5, 6] respectively. We then pass these arrays as arguments to the mean
function along with the axis
parameter set to 0, indicating that we want to calculate the mean across the corresponding elements of the arrays. Finally, we print the mean of the arrays.
Output:
Mean of Arrays: [2.5 3.5 4.5]
As we can see, the mean of the arrays [1, 2, 3] and [4, 5, 6] across the corresponding elements is [2.5, 3.5, 4.5].
Using Numpy Average with Multiple Arrays
Similarly to the mean
function, we can also use the average
function to calculate the average of multiple arrays. The process is the same as described in the previous section, where we pass the arrays as arguments to the average
function.
Let's consider an example to illustrate how to use the average
function with multiple arrays:
import numpy as np array1 = np.array([1, 2, 3]) array2 = np.array([4, 5, 6]) average_arrays = np.average([array1, array2], axis=0) print("Average of Arrays:", average_arrays)
In this example, we create two Numpy arrays array1
and array2
with values [1, 2, 3] and [4, 5, 6] respectively. We then pass these arrays as arguments to the average
function along with the axis
parameter set to 0, indicating that we want to calculate the average across the corresponding elements of the arrays. Finally, we print the average of the arrays.
Output:
Average of Arrays: [2.5 3.5 4.5]
As we can see, the average of the arrays [1, 2, 3] and [4, 5, 6] across the corresponding elements is [2.5, 3.5, 4.5].
Comparison: Numpy Mean vs Numpy Average
Both the mean
and average
functions in Numpy can be used to calculate averages, but they have slight differences in functionality.
The mean
function calculates the arithmetic mean of the array or along a specified axis, without considering any weights. It is a simple and straightforward way to calculate the average.
On the other hand, the average
function allows us to include weights when calculating the average. This can be useful when certain elements of the array have more importance or significance than others. By specifying the weights
parameter, we can assign different weights to different elements, resulting in a weighted average.
In terms of performance, there is no significant difference between the mean
and average
functions. Both functions are highly optimized and efficient, allowing us to process large arrays and perform calculations quickly.
Related Article: Python Scikit Learn Tutorial
Additional Resources
- Numpy Average Function Documentation